Start state
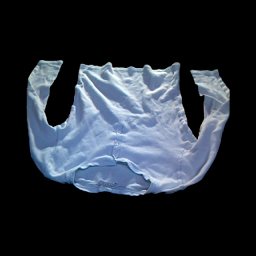
Planning in learned latent spaces helps to decrease the dimensionality of raw observations. In this work, we propose to leverage the ensemble paradigm to enhance the robustness of latent planning systems. We rely on our Latent Space Roadmap (LSR) framework, which builds a graph in a learned structured latent space to perform planning. Given multiple LSR framework instances, that differ either on their latent spaces or on the parameters for constructing the graph, we use the action information as well as the embedded nodes of the produced plans to define similarity measures. These are then utilized to select the most promising plans. We validate the performance of our Ensemble LSR (ENS-LSR) on simulated box stacking and grape harvesting tasks as well as on a real-world robotic T-shirt folding experiment.
*Contributed equally and listed in alphabetical order
Download Preprint
Our Method:
Available after paper acceptance
Available after paper acceptance