Start state
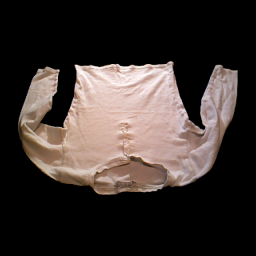
We present a framework for visual action planning of complex manipulation tasks with high-dimensional state spaces such as manipulation of deformable object. Planning is performed in a low-dimensional latent state space that embeds images. We define and implement a Latent Space Roadmap (LSR) which is a graph-based structure that globally captures the latent system dynamics. Our framework consists of two main components: a Visual Foresight Module (VFM) that generates a visual plan as a sequence of images, and an Action Proposal Network (APN) that predicts the actions between them. We show the effectiveness of the method on a simulated box stacking task as well as a T-shirt folding task performed with a real robot.
*Contributed equally and listed in alphabetical order
Download Paper
Our Method:
@inproceedings{lippi2020latent,
title={Latent Space Roadmap for Visual Action Planning of Deformable and Rigid Object Manipulation},
author={Lippi, Martina and Poklukar, Petra and Welle, Michael C and Varava, Anastasiia and Yin, Hang and Marino, Alessandro and Kragic, Danica},
booktitle={IEEE/RSJ International Conference on Intelligent Robots and Systems},
year={2020}
}
The code used to train the vae, build the graph in the latent space, and the action proposal network including all used hyperparameter can be found on the gitrepo:
Code Repository